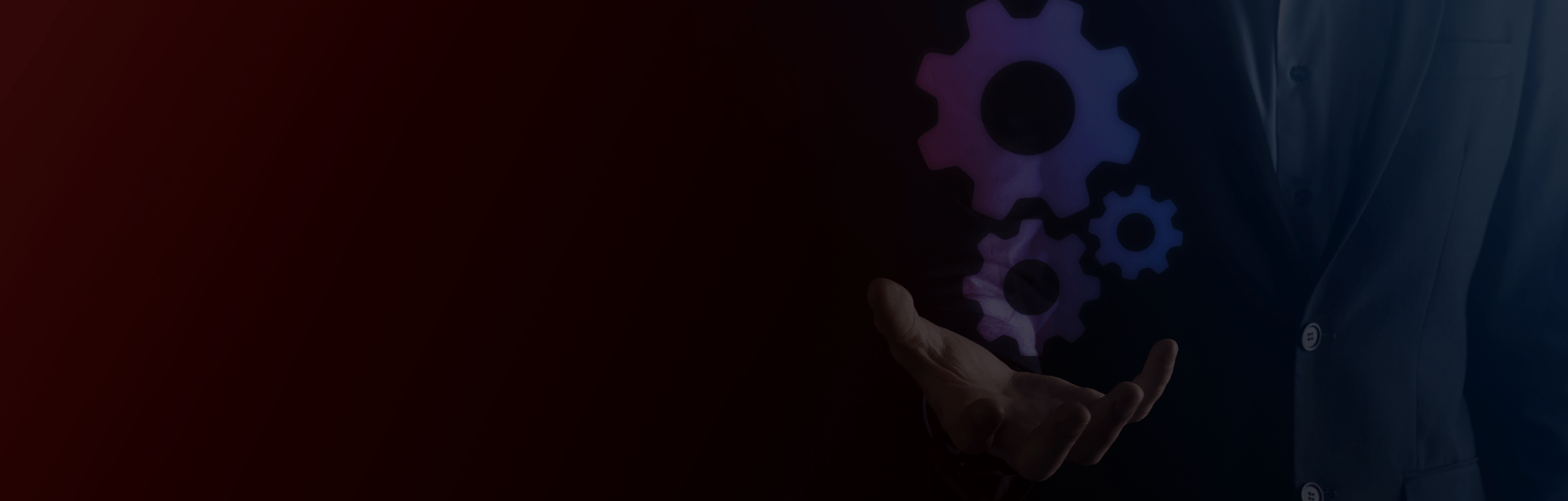
BIG DATA AND DATA ANALYTICS
Proposal engineering involves the preparation and submission of detailed proposals in response to requests for proposals (RFPs) from clients, which can include oil companies, government agencies, or other stakeholders. This process is critical for securing contracts for various projects, including exploration, drilling, production, and infrastructure development.
At Alpheratz, we are qualified to support in the following areas:
Big Data Technologies
Data Storage Solutions
- Data Lakes: Large repositories that store vast amounts of raw data in its native format until it is needed for analysis.
- Distributed File Systems: Technologies like Hadoop Distributed File System (HDFS) that enable the storage of data across multiple servers to enhance scalability and fault tolerance.
Data Processing Frameworks
- Apache Hadoop: An open-source framework for processing large datasets in a distributed computing environment.
- Apache Spark: A unified analytics engine that supports batch and real-time processing, providing faster data processing capabilities compared to traditional methods.
NoSQL Databases
- Types of Databases: Utilizing databases like MongoDB, Cassandra, and Redis that are designed to handle unstructured or semi-structured data, allowing for flexible data models and horizontal scaling.
Data Analytics Techniques
Descriptive Analytics
- Data Visualization: Using tools to create visual representations of data (charts, graphs) that help summarize historical data and trends.
- Reporting Tools: Generating regular reports to track key performance indicators (KPIs) and business metrics.
Predictive Analytics
- Statistical Modelling: Applying statistical techniques to identify patterns and predict future outcomes based on historical data.
- Machine Learning: Implementing algorithms that learn from data to make predictions or classifications, enhancing the ability to forecast trends.
Prescriptive Analytics
- Optimization Models: Using mathematical models to recommend actions based on data analysis, helping organizations make informed decisions.
- Simulation Techniques: Running simulations to assess the impact of different scenarios and strategies.
Data Integration and ETL (Extract, Transform, Load)
- Data Integration Tools: Technologies that combine data from different sources into a unified view, allowing for comprehensive analysis.
- ETL Processes: Extracting data from various sources, transforming it into a suitable format, and loading it into a data warehouse or analytics platform.
Data Governance and Management
- Data Quality Management: Ensuring that data is accurate, complete, and reliable through validation and cleansing processes.
- Metadata Management: Maintaining information about data assets, including data lineage, definitions, and usage.
Advanced Analytics and AI
- Natural Language Processing (NLP): Utilizing AI techniques to analyse and understand human language, often applied in sentiment analysis and chatbots.
- Deep Learning: Implementing neural networks to analyse complex data patterns, used in image recognition, speech recognition, and more.
Business Intelligence (BI)
- BI Tools: Utilizing software tools that help analyse data and present actionable information, such as Tableau, Power BI, and Qlik.
- Self-Service Analytics: Empowering business users to generate insights and reports without heavy reliance on IT, facilitating faster decision-making.
Cloud-Based Big Data Solutions
- Managed Services: Using cloud platforms (like AWS, Azure, or Google Cloud) that offer scalable big data solutions without the need for extensive on-premises infrastructure.
- Serverless Architectures: Implementing solutions that allow developers to run analytics without managing servers, simplifying deployment and scaling.
Industry Applications
- Customer Insights: Analysing customer behaviour and preferences to drive marketing strategies and improve customer experience.
- Operational Efficiency: Using data analytics to identify inefficiencies in operations, leading to cost savings and improved processes.
- Risk Management: Applying predictive analytics to identify and mitigate potential risks in various business areas.